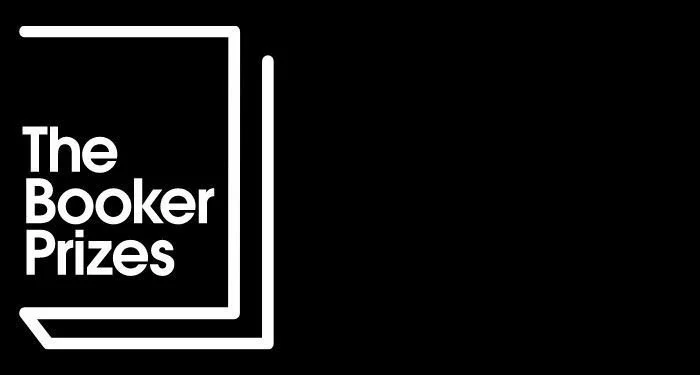
Topic Modelling The Booker Prize
This goal of this project was to see if there was anything to learn by text mining the Booker Prize shortlists over the the last 40 years and it formed the backbone of my masters thesis at UVA. I learned about the Hathi Trust tools after presenting the Historical Novel and Machine Learning project at the DH and University of Virginia Library Open House last fall.
I created a public collection of the books shortlisted and longlisted for the Booker Prize since 1969 using the Hathi Trust (which can be found here) and then adapted a short script written by staff at the Hathi Trust Research centre to download non-consumptive versions of the texts. With these in hand, I used the STM package to write an R script that would topic model the texts.
The Effect of Amazon on the Novel
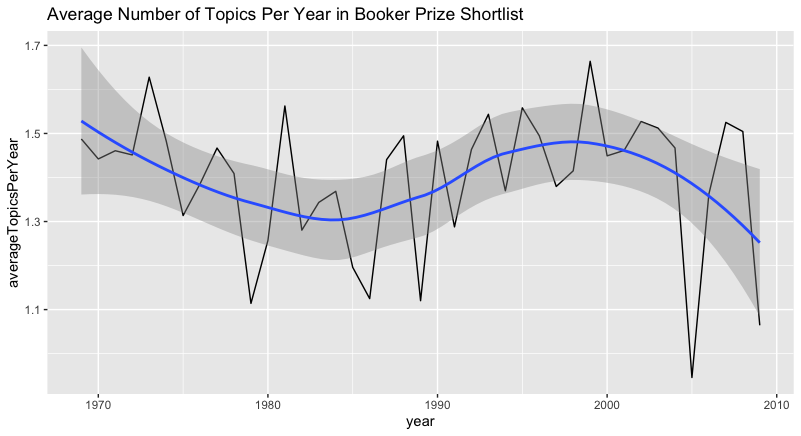
This graph counts the average number of topics per year found in the books shortlisted for the Booker Prize. In my thesis, I argued that this showed a steady increase in heteroglossia in the 80s and 90s, followed by a sharp decline in the 2000s. I defined heteroglossia as the way that topics competed for readers attention in a text. It is similar to the way that Mikhail Bakthin originally used the term but there are some differences. Whereas he is thinking about different voices working within a text, my interest is in the ways that different topics co-exist.
My research connects with Mark McGurl’s recent book, Everything and Less: The Novel in the Age of Amazon. He argues that Amazon has pushed the novel into two distinct versions of the form: the maximalist novel and minimalist novel. The former is grand in scope — a globe trotting spy thriller for example — whereas the latter is far narrower, more akin to a domestic romance. In my paper, I use the same polarities but, rather than seeing genre as the underlying structure like McGurl does, I use the number of topics (a maximalist novel contains several topics; a minimalist one only contains one or two). My data seems to show that the Booker Prize used to recognise topically maximal novels and has, since 2000, recognised increasingly topically minimal novels.
In my thesis, I argue that this is a direct consequence of Amazon’s presence in the British market. It particular, it is a result of algorithmic recommendation. The data suggests that the logic of Amazon was felt far earlier than previously thought.
The Influence of Tea on the English Novel
The graph above shows how closely the various topics in my analysis correlate. There are some that don’t feel very connected at all and others that correlate with a number of other topics. Topic 25, for example, which consists of novels by Thomas Keneally and Peter Carey (specifically The Confederates and The True History of the Kelly Gang) does not correlate with any other topic suggesting that the novels it contains are especially different from the rest of the novels examined.
The most connected topic is Topic 35. Its top 10 keywords include “table,” “drink,” “thought,” “tea,” “asked,” “room,” “glass,” “kitchen,” “put,” and “dinner.” The novels in Topic 35 include Mrs. Palfrey at the Claremont by Elizabeth Taylor, The Road to Lichfield by Penelope Lively and The Strange Case of Dr Simmons & Dr Glas by Dannie Abse.
One way of reading this graph would be to see that contents of Topic 35 correlate strongly with a large number of other topics in the Booker Prize texts. That might mean that drinking tea in a kitchen is central to a good number Booker Prize nominated novels but this needs further research to understand properly. This analysis did not make it into my thesis (which was only interested in Amazon) but it is intriguing and is something that I intend to follow up on.